Research Project of the Swiss National Science Foundation (SNSF)
Our Team
Cooperation between the American University of Sharjah, UAE and Bern Business School, Switzerland
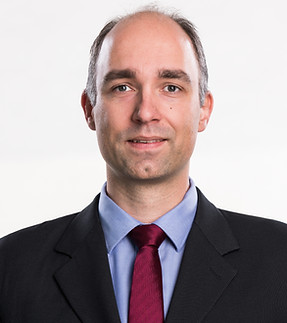
Joerg Osterrieder
Principal Investigator
Bern Business School, Switzerland
University of Twente, Netherlands​

Lennart John Baals
Team member
Bern Business School, Switzerland
University of Twente,
Netherlands
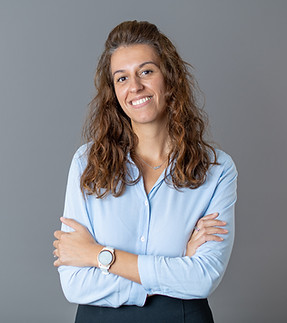
Branka Hadji Misheva
Team member
Bern Business School, Switzerland
​
​
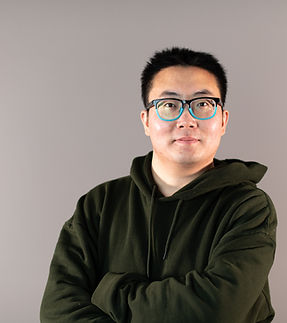
Yiting Liu
Team member
Bern Business School, Switzerland
University of Twente,
Netherlands
Description of the Research Project
Background: Peer-to-peer (P2P) lending has become an increasingly popular alternative to traditional bank lending, allowing individuals and businesses to borrow money directly from investors through online platforms without involving banks. While this method offers advantages such as higher returns for investors and greater access to credit for borrowers, it also brings unique risks. The decentralized nature of P2P lending means that loans are funded entirely by investors, without the safeguards of bank intermediation. In times of economic downturn, the risk is heightened as more borrowers who may not qualify for traditional bank loans turn to P2P platforms. This results in greater information asymmetry, higher default rates, and increased credit risk.
Rationale: The growing presence of P2P lending markets, especially during economic crises, exposes these platforms to significant risks, including adverse selection and moral hazard. Unlike traditional banks that use long-term relationships and extensive data to evaluate borrowers, P2P platforms have less detailed information and face higher levels of uncertainty. There is a critical need for robust credit risk models that can accurately assess the creditworthiness of borrowers in these markets and mitigate the risks for investors.
Overall objectives: This project aims to develop advanced, interpretable credit risk models tailored specifically to the needs of P2P lending markets. These models will address the unique challenges of P2P lending, such as higher information asymmetry, less regulation, and increased risk during economic downturns. The ultimate goal is to enhance trust between investors and P2P platforms by providing accurate tools for evaluating and mitigating credit risk.
Specific aims: The research will focus on understanding the key factors that contribute to credit risk in P2P lending, particularly during times of economic stress. It will develop new methods to model these risks, incorporating both static and dynamic factors to better predict potential defaults and mitigate losses for investors.
Methods: The project will develop credit risk models using network-based approaches, analyzing the connections between borrowers and lenders to identify patterns that indicate heightened risk. These models will be both static, looking at established risk factors, and dynamic, incorporating real-time data to adjust risk assessments as market conditions change.
Expected results: The research will produce new, cutting-edge credit risk models that can be applied directly to P2P lending platforms. These models will improve the ability of investors to assess borrower risk, leading to more accurate loan pricing, reduced default rates, and greater confidence in the P2P lending ecosystem.
Impact for the field: By providing more reliable credit risk models, this project will strengthen the P2P lending market, making it a more secure and viable alternative to traditional bank lending. The results of this research will be valuable not only to P2P platforms and investors but also to policymakers, financial institutions, and regulators who are interested in fostering safe and transparent lending environments.
Output and Results
This project has boosted collaboration both within the scientific communication and wider public. A few indicators to show the impact of this project are listed below.
2
Scientific Publications
2
Knowledge Transfer Events
1
Third-Party Funds
2
Datasets
2
Public Communications
3
Academic Events
2
Collaborations
Scientific Publications
01 Network centrality and credit risk: A comprehensive analysis of peer-to-peer lending dynamics
Liu, Y., Baals, L. J., Osterrieder, J., & Hadji-Misheva, B. (2024). Network centrality and credit risk: A comprehensive analysis of peer-to-peer lending dynamics. Finance Research Letters, 63, 105308.
This letter analyzes credit risk assessment in the Peer-to-Peer (P2P) lending domain by leveraging a comprehensive dataset from Bondora, a leading European P2P platform. Through combining traditional credit features with network topological features, namely the degree centrality, we showcase the crucial role of a borrower’s position and connectivity within the P2P network in determining loan default probabilities. Our findings are bolstered by robustness checks using shuffled centrality features, which further underscore the significance of integrating both financial and network attributes in credit risk evaluation. Our results shed new light on credit risk determinants in P2P lending and benefit investors in capturing inherent information from P2P loan networks.
02 Leveraging network topology for credit risk assessment in P2P lending: A comparative study under the lens of machine learning
Liu, Y., Baals, L. J., Osterrieder, J., & Hadji-Misheva, B. (2024). Leveraging network topology for credit risk assessment in P2P lending: A comparative study under the lens of machine learning. Expert Systems with Applications, 252, 124100.
Peer-to-Peer (P2P) lending markets have witnessed remarkable growth, revolutionizing the way borrowers and lenders interact. Despite the increasing popularity of P2P lending, it poses significant challenges related to credit risk assessment and default prediction with meaningful implications for financial stability. Traditional credit risk models have been widely employed in the field of P2P lending; however, they may not be capable to capture latent factor information inherent to a loan network based on similarity distances. Thus, in this study we propose an enhanced two-step modeling approach for Machine Learning (ML) that utilizes insights from network analysis and subsequently combines derived network centrality metrics with traditional credit risk factors to improve the prediction accuracy in the credit default prediction process. Through a comparative analysis of three classical ML models with varying degrees of complexity, namely Elastic Net (EN), Random Forest (RF), and Multi-Layer Perceptron (MLP), we showcase novel evidence that the systematic inclusion of network topology features in the credit scoring process can significantly improve the prediction accuracy of the scoring models. Additional robustness tests via the inclusion of randomly shuffled centrality metrics in the analysis, and a further comparison of the graph-based models against a pertinent state-of-the-art credit scoring model in form of XGBoost, further confirm our results. The insights from this study bear valuable conclusions for P2P lending platforms to further improve their scoring systems with graph-enhanced metrics, thereby reducing default risk and facilitating greater access to credit.
Academic Events
The team has received invitations to numerous international conferences, serving roles as keynote speakers, session chairs, or even organizing the events themselves.

8th Bern Conference
2024
September 29th, 2024. Dr. Hadji-Misheva was invited to talk as speaker at an inaugural research conference on Fintech and AI in Finance at the Department of Business, Bern University of Applied Science. The talk titled 'Leveraging Network Topology for Credit Risk Assessment' goes deeper into graph-theoretical concepts and their applications for research initiatives, advancements, and innovations in the field of credit risk scoring.
Collaborations
Department of Mathematics, American University of Sharjah, UAE
Prof. Dr. Stephen Chan
- In-depth constructive exchanges on approaches, methods, or results
- Publications
- Exchange of personnel
Department of Mathematics, University of Manchester, UK
Dr. Yuanyuan Zhang
- In-depth constructive exchanges on approaches, methods, or results
- Publications
Department of Mathematics, Renmin University, China
Prof. Dr. Jeffrey Chu
- In-depth constructive exchanges on approaches, methods, or results
- Publications
​
Department of Business, Bern Business School, Switzerland
Prof. Dr. Branka Hadji Misheva
- In-depth constructive exchanges on approaches, methods, or results
- Publications
- Exchange of personnel
​
COST Action CA19130 Fintech and Artificial Intelligence in Finance
Action Chair: Joerg Osterrieder
- In-depth constructive exchanges on approaches, methods, or results
- Publications
- Exchange of personnel
​
MSCA Industrial Doctoral Network on Digital Finance
Coordinator: Joerg Osterrieder
- In-depth constructive exchanges on approaches, methods, or results
- Publications
- Exchange of personnel
Third-Party Funds
The team has applied to and acquired several large national and international research funds, from institutional grants, national funding organisations, the Swiss National Science Foundation annd the Horizon Europe framework programme.
01
Leading House Asia: 2023 Call for Applied Research Partnerships, ETH
Bern University of Applied Science, CH
Proposal Number: FRG24-E-S25
Grant Period: 1 June 2024 – 31 May 2025
Title: From Digits to Dollars: The Evolution of Price Impact in Digital Assets
Yiting Liu (PI); Joerg Osterrieder(Co-PI)
Awarded: 50,000 CHF
02
SNSF Mobility Grant 2024 / 1
SNSF Mobility Research Grant 2024, CH
Bern University of Applied Science, CH
Proposal Number: 100018E_205487 / 3
Grant Period: 1 February 2024 – 31 August 2024
Title: Network-based credit risk models in P2P lending markets
Lennart John Baals (PI); Joerg Osterrieder(Co-PI)
Awarded: 20,000 CHF
03
SNSF Mobility Grant 2024 / 2
SNSF Mobility Research Grant 2024, CH
Bern University of Applied Science, CH
Proposal Number: 100018E_205487 / 2
Grant Period: 1 February 2024 – 31 August 2024
Title: Network-based credit risk models in P2P lending markets
Yiting Liu (PI); Joerg Osterrieder(Co-PI)
Awarded: 20,000 CHF